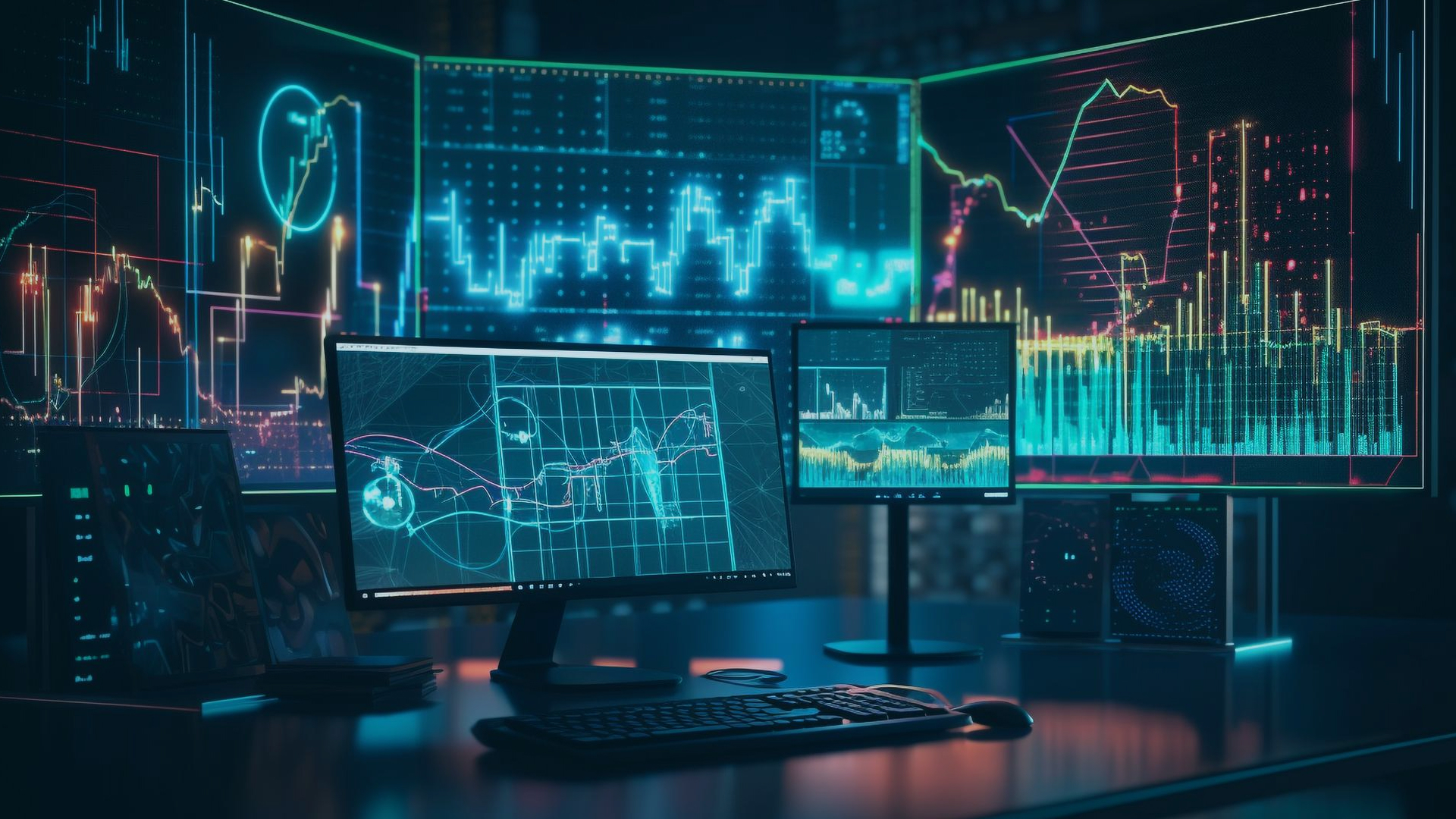
Machine Learning Approach: Data-Centric vs Model-Centric
Model-centric and data-centric Machine Learning are two different approaches that can be used in Artificial Intelligence. The Model-Centric approach consists of focusing on using the right set of algorithms, programming language and platform. Instead, the focus of the Data-Centric approach shifts to obtaining high-quality data.
Experts in the field are divided between those who prefer a Data-Centric and those who prefer a Model-Centric approach. In Machine Learning, there is no right or wrong approach, it is evaluated which one to use according to one’s strategic asset and thus the goals intended to be achieved.
Model-centric or data-centric approach: what to choose in Machine Learning? Let us try to understand the difference between the two approaches.
Data-Centric Approach in Machine Learning
The data-centric approach focuses on all actions aimed at curating the data: qualitative selection, labelling, monitoring, updating and management.
The goal of this approach is to produce the best data set to feed a given Machine Learning model and thus obtain an accurate result.
Therefore, the Data-Centric approach focuses on obtaining the right kind of data that are used to build high quality and high performance machine learning models.
Machine Learning and Model-Centric Approach
The model-centred approach aims to generate the best Machine Learning model for a given dataset. The idea behind it is to constantly work on and improve the model in order to achieve more effective performance.
Those who choose a model-centric approach therefore decide to focus on the model architecture and the learning process.
Machine Learning at Omninext
At Omninext we use a Data Centric approach that allows us to carry out data collection and engineering in order to create an accurate and goal-oriented business operation system with a constantly updated dataset and thus an artificial intelligence model that is always representative of reality.
Discover more: https://omninext.it/en/machine-learning.html